What Is Your Phone “Saying” Behind Your Back?
Introduction
User authentication has been for many years based on something the user knows in secret, such as a PIN or a swipe pattern, or on something the user has, like an ID card. These were the most popular methods, but numerous studies showed that these systems did not provide sufficient convenience and security, especially on mobile devices. These drawbacks led to the need for new authentication methods. Thus, biometric methods emerged, based on something that the user is or how they perform something. These biometric systems are based on the use of physiological data of the user (e.g. fingerprint, face, vein map, etc.) or behavioural data (e.g. gait, touchscreen, keystroke, etc.).
Behavioural biometrics are one of the most studied authentication methods in recent years. This is because they do not require the user to perform any specific action, for example, gait biometrics is a method based on behavioural data. It consists of identifying a person according to their walking characteristics. As mobile devices advance in technology, embedded sensors can be used to more accurately capture this biometric behavioural data (e.g. accelerometer, gyroscope, compass).
The main problem is that the data extracted by the sensors of mobile devices can contain a large amount of personal and sensitive information such as gender, age and ethnicity, health parameters or even the activity the user is performing. The main reason why this data can be extracted is that different physiological and behavioural traits affect the handling patterns of smart devices. For example, a taller person will need to take fewer steps than a shorter person to perform the same action.
Thus, if this data is to be used to verify a person, it can also distinguish the activity performed by the user or even their physiological traits. Therefore, this technology is considered as a possible source of invasion of personal privacy and this sensitive data must be protected.
Sensitive Data by definition in the GDPR
The European Union has provided the General Data Protection Regulation (GDPR) which defines sensitive data as a subset of personal information, that includes: i) personal data revealing racial or ethnic origin, political opinions, religious or philosophical beliefs; ii) trade-union membership; iii) genetic data, biometric data processed solely to identify a human being; iv) health-related data; and v) data concerning a person’s sex life or sexual orientation.
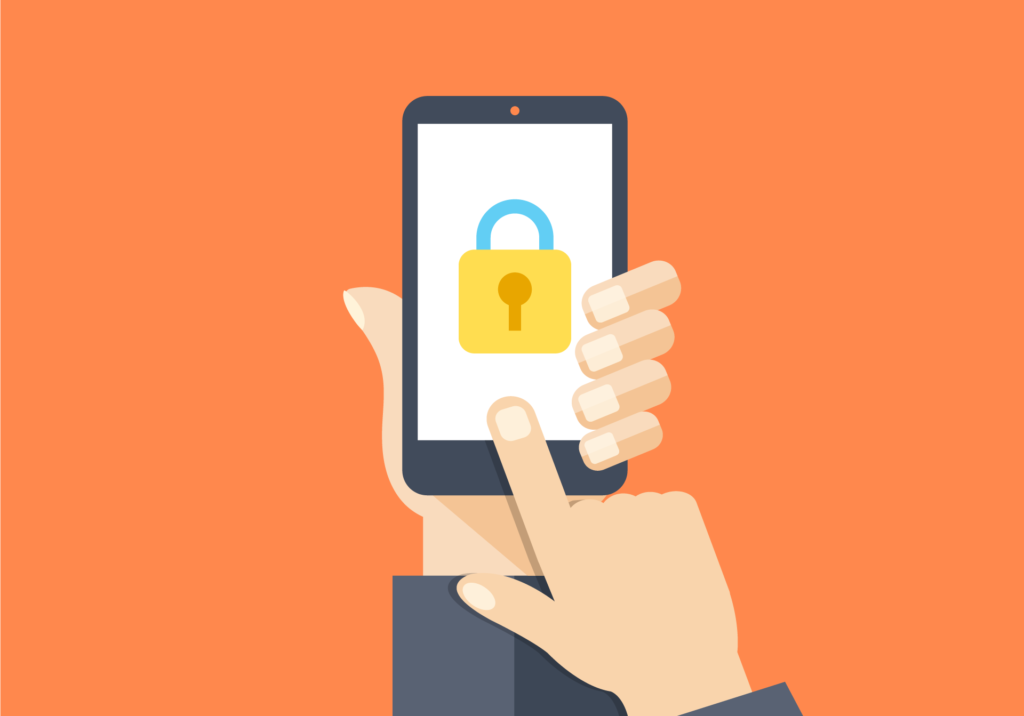
User profiling is any form of automated processing of personal data. This technique can quickly and efficiently extract sensitive attributes of users through their interaction with smart devices. Following the GDPR, “the data subject should have the right not to be subject to decision”, including measures or evaluating personal aspects among others. The main problem is that no specific permission is needed to access the information from these sensors, which reduces users' privacy and security.
The data subject should have the right not to be subject to a decision, which may include the assessment of personal aspects relating to him or her, which is based solely on automated processing and which produces legal effects concerning him or her or similarly significantly affects him or her, such as the automatic refusal of an online credit application or e-recruitment practices without human intervention.
Based on these statements in the GDPR, it is important to know some of the sources of sensitive information within mobile device sensors and what types of sensitive data can be acquired from each of these sources.
Sources of invasion of sensitive data
Some of the sensitive attributes that can be extracted from sensors of smart devices include: Demographics, Activity and behaviour, health parameters and body features, mood and emotion, location, keystrokes and text.
Demographics
Demographics can be all the information related to gender, age, ethnicity, political opinions or sexual orientation among others. For example, it is possible to infer a subject's age while performing a task on the screen. The traits one has at different ages affect the way one performs that activity.
Some other sources from which such information can be acquired include touchscreen or usage patterns like geo-tagged photos on social networks.
Activity and Behaviour
The activity the user is doing or the normal behaviour during the day is another important information that can be extracted from mobile sensor data. From accelerometer or gyroscope data it is possible to identify the activity a subject is doing. Another source of privacy invasion can be GPS data. By having the information about the geographic positioning of a subject at any given moment, it is possible to extract the type of activity a subject is doing with simple mathematical operations.
Health Parameters and Body Features
BMI (Body Mass Index) can be calculated from two body parameters of a person: height and weight. These two parameters are considered demographic information but, after calculating the BMI, it is possible to infer many health parameters. From the accelerometer data it is easy to extract these body characteristics. In addition, stress can be extracted from the accelerometer. Parkinson's disease can also be identified by hand movements or keystroke patterns.
Another important source of information can be geolocation, for example, it is possible to detect Bipolar Disorder (BD) according to the geographical location of a user's location.
Mood and Emotion
A person's mood can be inferred from how he or she performs an activity. An activity is not performed with the same efficiency, effort or motivation when the subject is in a bad mood. One example of this is that, based on data from a smartwatch's motion sensors, when a user performs the same activity, it is possible to differentiate whether they are in a happy, neutral or sad mood. When a person is in a happier mood, he or she performs the activity with more enthusiasm and eagerness. As the mood worsens, so do these traits.
Another example is how different game modes in a video game affect the user. For example, from how the user interacts with the screen, it is possible to tell whether the user is stressed, relaxed, frustrated or bored.
Location
Apart from the known geolocation sensors (GPS), studies show how it is possible to extract a person's location using other sensors. One example is motion sensors (accelerometer, gyroscope and magnetometer). It can also be inferred from the different Wi-Fi networks to which a user's device is connected.
Keystroke and Text
Other information that can be acquired are passwords and PINs. There are applications created to determine the region of the screen that the user is touching from data extracted by the device's motion sensors (accelerometer and gyroscope).
Conclusion
After all this collected information, it can be seen that invading a user's privacy and extracting their sensitive data is not a complicated task from a technical point of view. Therefore, apart from different laws, such as the GDPR, it is necessary to approach this problem from a technical point of view.
The aim is to ensure that, even if an attempt is made to attack a system and circumvent the law, it is possible to protect any user and achieve a high level of privacy. Researchers are therefore working on privacy protection methods. One example is GaitPrivacyON, a mobile walk verification approach that considers privacy-preserving methods [3]. It provides accurate authentication results while preserving the privacy of the subject. After showing all the possible sources of invasion and the different sensitive data that can be inferred, a multidisciplinary study of the field and further research is needed.
Recommended resources:
[1] EU 2016/679 (General Data Protection Regulation), https:// gdpr-info.eu/.
[2] P. Delgado-Santos, G. Stragapede, et al. "A Survey of Privacy Vulnerabilities of Mobile Device Sensors." arXiv preprint arXiv:2106.10154 (2021), https://arxiv.org/pdf/2106.10154.pdf
[3] P. Delgado-Santos, et al. "GaitPrivacyON: Privacy-Preserving Mobile Gait Biometrics using Unsupervised Learning." arXiv preprint arXiv:2110.03967 (2021), https://arxiv.org/pdf/2110.03967.pdf
This blogpost was written by Paula Delgado-Santos. She received the M.Sc. degree in Telecommunications Engineering from Universidad Autonoma de Madrid, Spain, in 2020. At the same time, she was working in a scholarship of IBM. In 2019/2020 she was working at a Swiss University, HEIG-VD, as a Data Scientist. In 2020 she started her PhD with a Marie Curie Fellowship within the PriMa (Privacy Matters) EU project at University of Kent, United Kingdom. Her research interests include signal and image processing, pattern recognition, machine learning, biometrics and data protection.
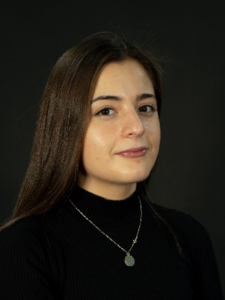