Soft biometric privacy and fairness: two sides of the same coin?
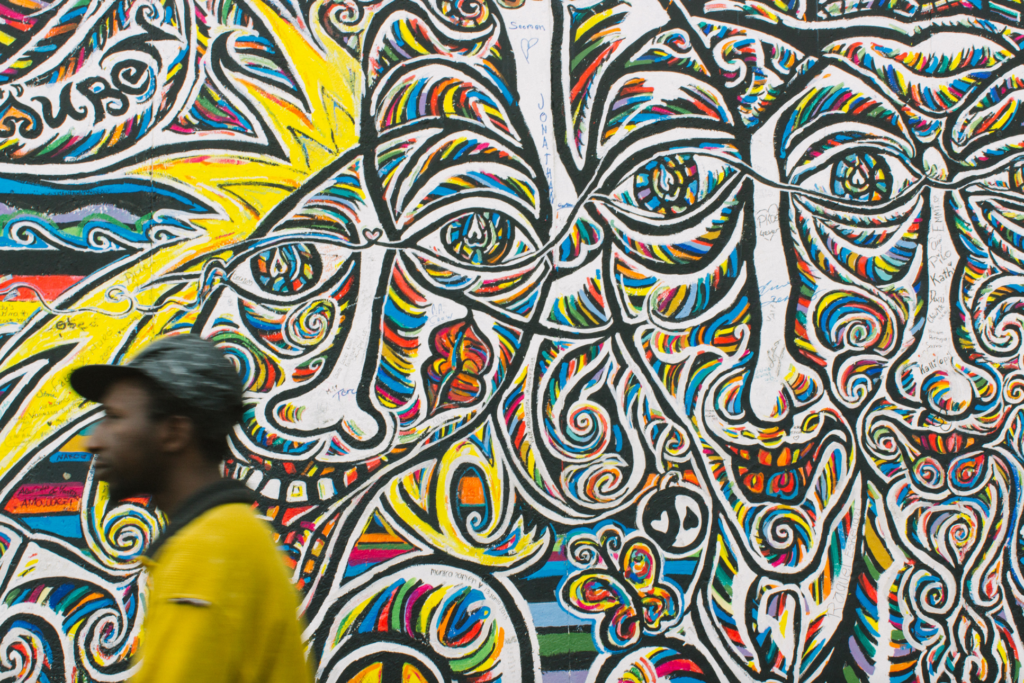
Many people associate the processing of facial images with face recognition, but actually, a lot of other information can be extracted from faces: from usual demographics such as age or gender to more sensitive and consequential information, like health condition or job suitability. Naturally, this raises serious legal and ethical concerns about data processing, such as algorithms being deemed a reliable source for important decision-making that can have a harmful influence on people's lives.
There have been several events that caught a lot of attention for the issues mentioned, such as the reliance on an inaccurate and biased face recognition model to identify criminals that led to the arrest of Robert Julian-Borchak Williams, an African American man although he was not guilty. Because of the racial imbalance inherent in the data that was used to train the model, the algorithm was implicitly biased against people with darker skin tones.
Another debate erupted after a group of researchers claimed that they developed an algorithm that could reliably predict criminality from facial images. Much of the criticism focused on the fact that there is no guarantee that such a system will not exacerbate existing prejudice and bigotry towards specific groups.
The idea of evaluating crime from a facial image, as well as the reality that it is inherently biased, is enough to make anyone's moral compass sway. On the one hand, it makes us consider what we should even allow in terms of data processing, but it also makes us consider to what extent we should trust an automated decision in general if we know that it might be severely biased against particular groups. In essence, it boils down to two factors: Soft biometric privacy and fairness.
Fairness and soft biometric privacy in a nutshell
Soft biometric attributes are a set of traits that can be used to classify people into groups but do not necessarily single out, i.e identify individuals. In facial biometric applications, these traits tend to be physical such as ethnicity or biological sex. They can also be more specific like skin color and hair type. Soft biometric privacy in the case of facial images or templates, aims to prevent the inference of one or more of these soft biometric attributes while preserving some desired information (utility). For instance, in my PriMA PhD project I strive to remove as many soft biometric attributes from facial images and templates as possible while still allowing a recognition algorithm to verify the identity of subjects.
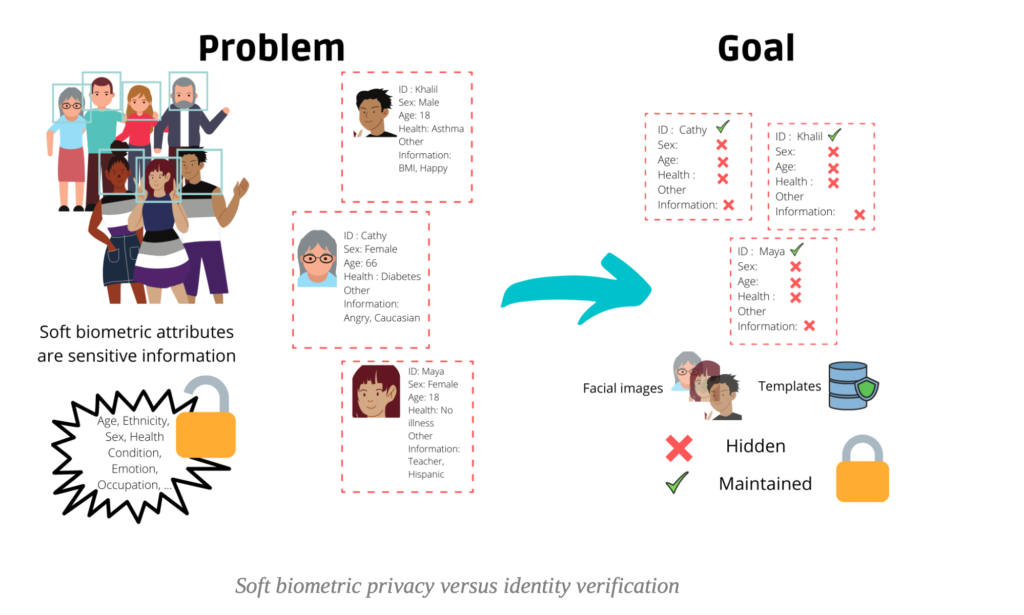
Fairness on the other hand, even though by its name it describes a commonly understood moral value, is more complex. There are two main paradigms to define fairness: group fairness and individual fairness.
Essentially, group fairness is based on the idea that an algorithm should have equal proportions of demographic subgroups in each of the predicted classes for it to be called fair.
For instance, if a bank uses an algorithm to predict if potential credit clients are financially solvent, the system will be "group fair" if it does not predict that one demographic group (e.g women) will be more financially defective than the other (e.g men).
Individual fairness, on the other hand, is based on the idea of treating similar persons for a certain categorization task similarly in terms of the probability distributions of the outcomes. For example, if two people have a similar amount of income and they each apply for loans with similar values but one gets the approval and the other doesn’t, then we have a case of individual unfairness.
Both fairness models as well as privacy methods have their limitations but provide foundations for tackling impending problems.
What do soft biometric privacy and fairness have in common?
Admittedly, traits that are prone to privacy violation are also likely to be the object of bias. For instance, commercial face verification systems may generate templates that can be further trained for classifying whether a person is male or female . This makes such systems privacy-invasive since they are only supposed to perform identity verification. On top of that, they also do not work as well for women as for men which makes them biased.
One of the major disadvantages of group fairness is that attempting to ensure fairness between different groups of a demographic variable such as age, does not guarantee fairness between subgroups of each group. The more we describe a person, the more detailed categories we can assign to them, and the more sparse these categories become.
Soft biometric privacy has a similar issue in that the definition of such variables is very fluid and can vary depending on socio-cultural contexts. As a result, implementing a privacy solution based on an arbitrary definition of demographic variables is unlikely to be viable if a person targets the same variables but with the categories defined differently.
While individual fairness tries to compensate for the group's shortcomings, it also looks to be difficult to implement in practice. As it tries to move away from the reliance on predefined categories, it becomes highly dependent on the task considered. In fact, choosing a similarity measure between individuals for every task is far from being trivial in the same way that quantifying privacy is challenging when we consider a realistic attack scenario. In general, an adversary could be interested in anything, from age to health-related cues or any other characteristic that is possible to infer automatically from faces. Without making assumptions on how persistent an adversary is and what kind of information he is interested in, how would we decide if a set of images or templates are private enough?
Soft biometric privacy as a path to fairness
Beyond the elements in common described above, soft biometric privacy may have the potential to enhance fairness.
Assuming we have an algorithm that is designed for a specific task, such as financial solvability prediction, that we would like to keep private with respect to a set of sensitive attributes such as biological sex or age, we would expect that the features selected as relevant for the decision-making process do not allow us to distinguish between the groups of such attributes.
When considering biological sex as an example, a private version of a face verification system would only select features that would retain a similar distribution for males and females while still achieving good performance. Naturally, this is consistent with the goal of group fairness; if the features used do not distinguish between males and females, there will most likely be similar error rates in both groups.
The research fields of soft biometric privacy and fairness are now growing in parallel, and this is expected to keep going on. Whether it is possible to obtain sufficient guarantees for either one of them while maintaining a state-of-the-art recognition performance remains an open research question. As a result of the numerous points of convergence they share, there is the possibility that an intersectional approach between the two could yield some quite intriguing discoveries.
This blogpost was written by Zohra Rezgui. Since 2020, she is a PhD candidate at the University of Twente. Her research within PriMa aims to mitigate biometric profiling in facial images and templates.
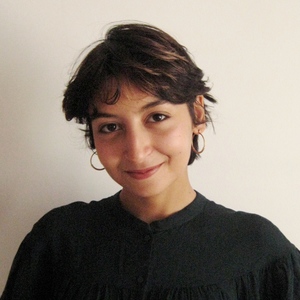