Can Adversarial Attacks Protect Facial Privacy?
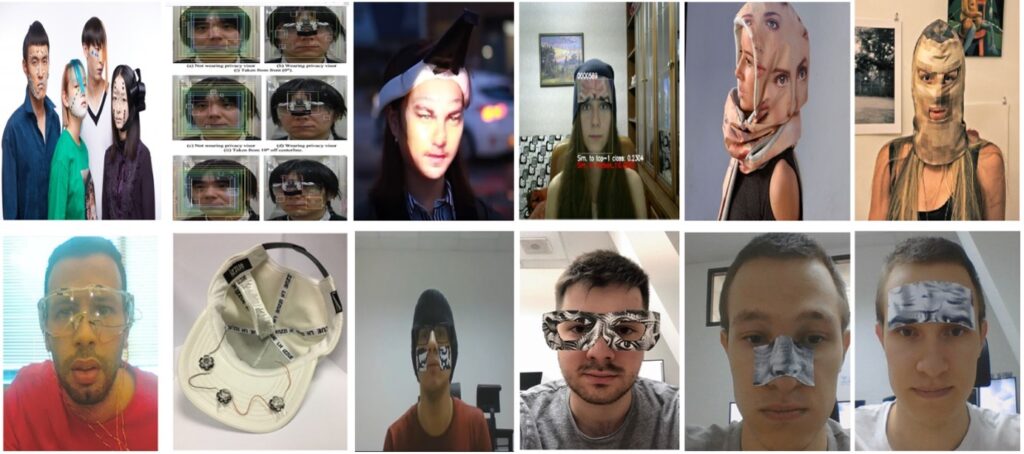
Figure 1: Facial privacy protection methods at presentation-level
Adversarial Attacks
Adversarial attacks are a type of computer security threat that involves intentionally modifying data inputs to cause errors in machine learning algorithms. In the case of face recognition systems, adversarial attacks can be used to modify images or videos of a person's face in a way that confuses the system, causing it to either fail to recognize the person or misidentify them.
One common type of adversarial attack is to add subtle perturbations to the input image, which are not easily perceptible to the human eye but can cause the recognition algorithm to fail. These perturbations can be generated using machine learning algorithms themselves, or by using hand-crafted methods.
Another type of adversarial attack involves creating "adversarial faces" that are designed to intentionally confuse FR systems. These adversarial faces are created by manipulating facial features in a way that makes them difficult for the recognition algorithm to process. For example, an adversarial face might have eyes that are slightly further apart than usual or a mouth that is slightly wider or narrower than normal.
Adversarial attacks can be used by individuals to protect their privacy in situations where FR technology is being used without their consent or knowledge. For example, if a store is using face recognition to track customer behaviour, an individual could wear makeup or a mask that includes adversarial perturbations, making it difficult for the system to track their movements.
Similarly, adversarial attacks can be used by activists or others who are concerned about the use of face recognition technology in law enforcement or surveillance. By creating adversarial faces or modifying images in a way that confuses recognition algorithms, activists can make it more difficult for authorities to identify individuals who are participating in protests or other activities. A recent article by Vakhshiteh et al. [2] discussed about most of the major techniques used for adversarial attacks against FR systems. Adversarial attacks are not only used for privacy protection systems but also to evaluate the vulnerabilities of machine learning-based biometric systems.
Limitations
There are, of course, potential drawbacks to using adversarial attacks for facial privacy protection. For example, it may be difficult to ensure that the adversarial perturbations or faces are effective across a wide range of recognition systems, which could limit their usefulness in practice. Additionally, some people may find it uncomfortable or inconvenient to wear makeup or masks designed to confuse recognition systems.
In conclusion, adversarial attacks on FR systems can provide a powerful tool for protecting facial privacy in a world where FR technology is becoming increasingly ubiquitous. By using these attacks to intentionally confuse recognition algorithms, individuals can limit the ability of others to track their movements or identify them in images and videos. While there are potential drawbacks to using adversarial attacks for facial privacy protection, the benefits of these techniques are clear, and they are likely to play an increasingly important role in privacy protection in the years to come.
[1] Hasan, M.R., Guest, R., Deravi, F.: Presentation-Level Privacy Protection Techniques for Automated Face Recognition - A Survey. ACM Comput. Surv. (2023).
[2] Vakhshiteh, F., Nickabadi, A., Ramachandra, R.: Adversarial Attacks Against Face Recognition: A Comprehensive Study | IEEE Access, vol. 9, pp. 92735-92756, (2021), .
This blogpost was written by Rezwan Hasan. Since October 2020, he has been working as a Marie Curie Early-Stage Researcher at the School of Engineering of the University of Kent, UK. His research within the PriMa ITN Horizon 2020 project aims to investigate and develop emerging and future approaches to sensor-level facial identity hiding.
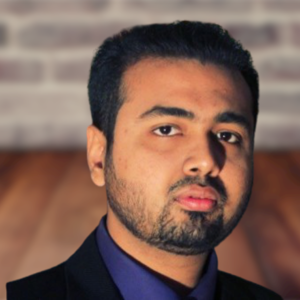