Today’s debate over biometric data:
a 5-minute non-technical compendium
Introduction
Humans can attempt to identify other humans in many ways. The simplest and most common way is probably by looking at their face, if they are close enough, or their entire figure, from distance. Perhaps their decision can be based on the style of clothes they are wearing. If they cannot rely on sight, they can recognize each other’s voice, if previously heard for a few times. But there is more. If a family member walks downstairs, you are probably going to recognize them from the sound of their steps, based on attributes your brain has immediately extracted from the bare sound, such as their pace or an estimate of their weight. If the bell rings and someone routinely comes back around that time, you might possibly predict who is at the door. Often, you can recognize your regular desk mate’s handwriting, even without paying attention to the characters written. A similar mechanism applies to machines, in a way.
Definitions, first
Biometric techniques encompass all technologies or operations that rely on specific technical processing of data relating to physiological or behavioral aspects of the human body. A traditional classification of biometric traits is based on whether a particular characteristic is a biological trait, such as the face, the iris, or the fingerprint. This category is also referred to as physiological. On the other hand, all means that enable or contribute to differentiating between individuals throughout the way they perform activities are labelled as behavioral. Examples of behavioral biometrics include voice, gait, routine patterns, handwriting, etc.
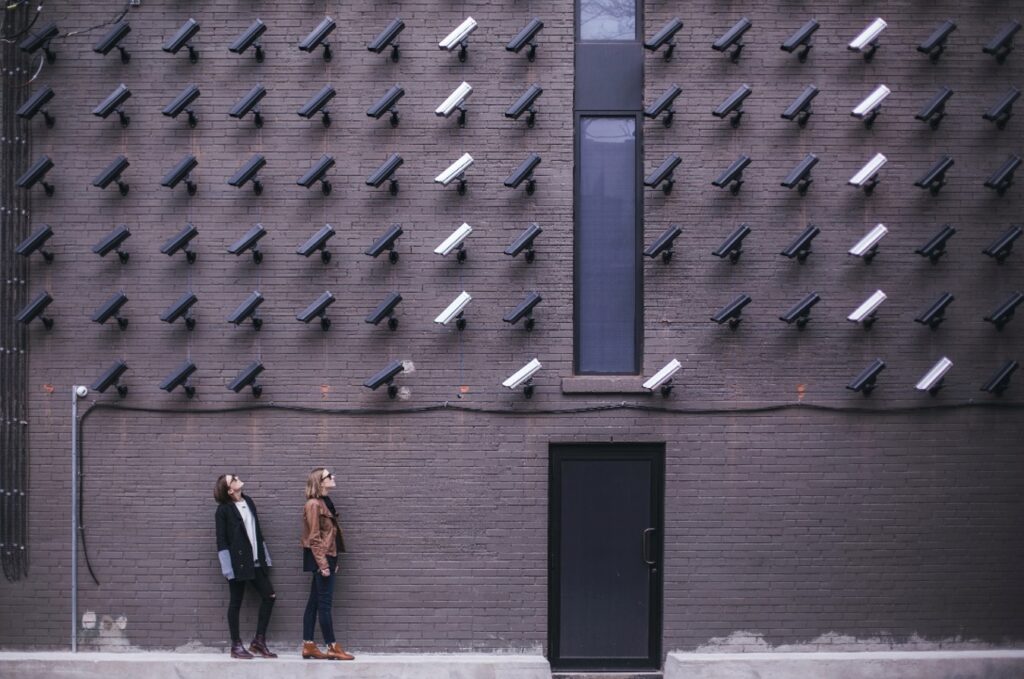
Biometric data can be exploited for many different applications. Some of the most popular ones include the following:
(i) Authentication/identification of human individuals. For this purpose, traditionally it was possible to achieve higher recognition accuracy with physiological biometrics. Consequently, authentication systems deployed today (in passports, for instance, or smartphones) are mainly based on physiological biometrics such as fingerprint or face.
(ii) Categorization of human individuals. Considering permanent or long-term characteristics, recent research has explored the possibility of extracting personal attributes, such as gender, age, ethnicity, hair color, height, weight, and so on from all kinds of biometric traits. These personal attributes are called soft biometrics. Such information, while not necessarily unique to an individual, can be used in a variety of applications. For instance, it can be used in conjunction with physiological biometric traits to improve recognition performance (e.g., fusing face with gender information), or to generate qualitative descriptions of an individual (e.g., young Asian female with dark eyes and brown hair).
(iii) Detection of temporary or permanent conditions of a human individual. Examples include sentiments such as fear, fatigue, or illness. The main ethical issues raised by the biometric detection of human conditions follow from its potentially intrusive nature, often analyzing very intimate traits, some of them beyond the individual’s consciousness.
Our project, PriMa, is focused on the analysis and mitigation of privacy risks in our rapidly digitalizing society. The possibility that acceptable privacy may become unattainable is concrete, unless technological and societal steps are taken to allow citizens to regain control of their personal information.
5 factors contributing to this scenario are described below.
1 - The magic of machine learning
“Any sufficiently advanced technology is indistinguishable from magic”, Arthur C. Clarke.
Nowadays, you do not need a job in the tech industry or academia to have heard the term “machine learning” (ML) in the last years. It is a sub-field of Artificial Intelligence that includes a variety of computer algorithms. American computer scientist Tom M. Mitchell provided for it a widely quoted definition: "A computer program is said to learn from experience E with respect to some class of tasks T and performance measure P if its performance at tasks in T, as measured by P, improves with experience E." In other words, by recognizing patterns lying within the data, computer programs learn information they were not explicitly provided with, becoming able to carry out specific tasks related to such data with better results.
ML has truly revolutionized the world, and its fields of applications are countless: from image processing and speech recognition to social media and product recommendation, including health care and autonomous driving (to name a few). Not surprisingly, also the performance of the processing of biometric data is not immune from the benefits of ML.
Perhaps the most fundamental aspect of ML is what Mitchell has defined as E: the data. This brings us to the second point.
2 - The ubiquity of sensing devices
The exponential growth of ML is related to the huge availability of data, due to the miniaturization of electronic circuits, allowing unprecedented sensing capabilities, processing power and memory in limited space, and to the increased bandwidth (maximum rate of data transfer).
Mobile devices such as smartphones, tablets, and wearables are provided with several sensors that can acquire, process, and transmit a vast amount of heterogeneous biometric data in a transparent way, in other words, without users noticing or having to actively do anything. The ubiquity of mobile devices (for instance, there were 3.9 billion smartphones globally in 2016, estimated to rise to 6.8 billion by 2022) and their always-on nature have turned this technology into a potential source of major invasion of personal privacy.
3 – The unawareness of people
People are generally unaware of what lies under the simple usage of a smartphone, from the point of view of the collection and usage of biometric data. Even for the experts it is difficult to grasp the complexity and the potential consequences of this scenario, let alone the general public.
4 – The commercial and political interests
Many industries are flourishing thanks to the technological advancement fueled by the computers’ ability to learn and to the availability of data. While on one hand we all undoubtedly benefit from it as, for many aspects, it has made our life more comfortable, efficient, and secure, this trend will inevitably keep calling for more data, including user personal and biometric data. Many services are today offered without any monetary cost if the user consents to share personal information. Without regulations, the risk is that privacy would become unachievable in the digital domain.
Additionally, starting from the observation that almost everyone constantly carries mobile devices constantly acquiring their personal and biometric data, such data could be exploited not only for commercial purposes, but also for political ones, to establish control over the population. In the 18th century, social theorist Jeremy Bentham designed a system of control called panopticon. The idea is to allow all prisoners of an institution to be observed by a single security guard, without being able to tell whether they are being watched. The inmates cannot know when they are being watched, and always act as they are, regulating their own behavior. Applied to today’s world, Bentham’s dystopic scenario could become concrete as all devices able to capture data and connected to the internet could effectively be used to track citizens.
5 – The complexity of regulating the digital world
Before rushing to the conclusion that we might end up living in Orwell’s 1984, efforts have been and are being carried out by governmental organs to prevent the digital world to resemble too closely a cyber far west. The European Union has provided the General Data Protection Regulation (GDPR), to protect citizens’ right with regard to their personal and sensitive data. Sensitive data has been defined as: i) personal data revealing racial or ethnic origin, political opinions, religious or philosophical beliefs; ii) trade-union membership; iii) genetic data, biometric data processed solely to identify a human being; iv) health-related data; and v) data concerning a person’s sex life or sexual orientation. Automated processing of user biometric data can easily reveal such attributes and the GDPR was designed also to address this. While the GDPR is a modern corpus globally regarded as the forefront of digital data protection, its enforcement in practice is a non-trivial, cross challenge for the experts. Additionally, in many areas of the world, such as Asia or the USA, similar effective regulations are laxer and not as homogeneous.
Conclusion
The digitalization of society has several advantages, including storage capacity, access and transmission speed, absence of time-related degradation of physical objects, among others, and with the ever-growing capacity of computers to automatically process data, it has become possible to mine structures and relationships lying in the data to extract information in unprecedented ways. We should look at it as a new technological revolution, which is shaping the world of tomorrow, in an extremely rapid and irreversible way. While it is duty of the experts to work to guarantee the citizen’s rights in the digital domain too, it is important, for the non-technical, to be informed and to participate to the necessary, yet stimulating, debate of privacy risks for the creation of a safe future.
Recommended resources:
[1] “Biometric Recognition and Behavioural Detection”, Study Requested by the JURI and PETI committees, Policy Department for Citizens’ Rights and Constitutional Affairs, European Parliament, https://www.europarl.europa.eu/RegData/etudes/STUD/2021/696968/IPOL_STU(2021)696968_EN.pdf
[2] A. Dantcheva, P. Elia and A. Ross, "What Else Does Your Biometric Data Reveal? A Survey on Soft Biometrics," in IEEE Transactions on Information Forensics and Security, vol. 11, no. 3, pp. 441-467, March 2016, doi: 10.1109/TIFS.2015.2480381.
[3] “The Social Dilemma”, https://www.netflix.com/es/title/81254224
[4] “Coded Bias”, https://www.netflix.com/es/title/81328723
[5] “Facial Recognition: Last Week Tonight with John Oliver (HBO)”, https://www.youtube.com/watch?v=jZjmlJPJgug&ab_channel=LastWeekTonight
[6] P. Delgado-Santos, G. Stragapede, et al. "A Survey of Privacy Vulnerabilities of Mobile Device Sensors." arXiv preprint arXiv:2106.10154 (2021), https://arxiv.org/pdf/2106.10154.pdf
This blogpost was written by Giuseppe Stragapede. He received his M.Sc. degree in Electronic Engineering from Politecnico di Bari, Italy, in 2019. After one year as a computer vision engineer in the industry, in 2020 he started his PhD with a Marie Curie Fellowship within the PriMa (Privacy Matters) EU project in the Biometrics and Data Pattern Analytics - BiDA Lab, at the Universidad Autonoma de Madrid, Spain. His research interests include signal processing, machine learning, biometrics and data protection.
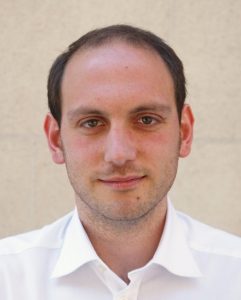